Relational IBL in classical music
Identifieur interne :
000151 ( PascalFrancis/Corpus );
précédent :
000150;
suivant :
000152
Relational IBL in classical music
Auteurs : Asmir Tobudic ;
Gerhard WidmerSource :
-
Machine learning [ 0885-6125 ] ; 2006.
RBID : Pascal:06-0438222
Descripteurs français
English descriptors
Abstract
It is well known that many hard tasks considered in machine learning and data mining can be solved in a rather simple and robust way with an instance- and distance-based approach. In this work we present another difficult task: learning, from large numbers of complex performances by concert pianists, to play music expressively. We model the problem as a multi-level decomposition and prediction task. We show that this is a fundamentally relational learning problem and propose a new similarity measure for structured objects, which is built into a relational instance-based learning algorithm named DISTALL. Experiments with data derived from a substantial number of Mozart piano sonata recordings by a skilled concert pianist demonstrate that the approach is viable. We show that the instance-based learner operating on structured, relational data outperforms a propositional k-NN algorithm. In qualitative terms, some of the piano performances produced by DISTALL after learning from the human artist are of substantial musical quality; one even won a prize in an international 'computer music performance' contest. The experiments thus provide evidence of the capabilities of ILP in a highly complex domain such as music.
Notice en format standard (ISO 2709)
Pour connaître la documentation sur le format Inist Standard.
pA |
A01 | 01 | 1 | | @0 0885-6125 |
---|
A03 | | 1 | | @0 Mach. learn. |
---|
A05 | | | | @2 64 |
---|
A06 | | | | @2 1-3 |
---|
A08 | 01 | 1 | ENG | @1 Relational IBL in classical music |
---|
A11 | 01 | 1 | | @1 TOBUDIC (Asmir) |
---|
A11 | 02 | 1 | | @1 WIDMER (Gerhard) |
---|
A14 | 01 | | | @1 Austrian Research Institute for Artificial Intelligence @2 Vienna @3 AUT @Z 1 aut. |
---|
A14 | 02 | | | @1 Department of Computational Perception, Johannes Kepler University Linz, and Austrian Research Institute for Artificial Intelligence @2 Vienna @3 AUT @Z 2 aut. |
---|
A20 | | | | @1 5-24 |
---|
A21 | | | | @1 2006 |
---|
A23 | 01 | | | @0 ENG |
---|
A43 | 01 | | | @1 INIST @2 21011 @5 354000133342720010 |
---|
A44 | | | | @0 0000 @1 © 2006 INIST-CNRS. All rights reserved. |
---|
A45 | | | | @0 19 ref. |
---|
A47 | 01 | 1 | | @0 06-0438222 |
---|
A60 | | | | @1 P @2 C |
---|
A61 | | | | @0 A |
---|
A64 | 01 | 1 | | @0 Machine learning |
---|
A66 | 01 | | | @0 NLD |
---|
C01 | 01 | | ENG | @0 It is well known that many hard tasks considered in machine learning and data mining can be solved in a rather simple and robust way with an instance- and distance-based approach. In this work we present another difficult task: learning, from large numbers of complex performances by concert pianists, to play music expressively. We model the problem as a multi-level decomposition and prediction task. We show that this is a fundamentally relational learning problem and propose a new similarity measure for structured objects, which is built into a relational instance-based learning algorithm named DISTALL. Experiments with data derived from a substantial number of Mozart piano sonata recordings by a skilled concert pianist demonstrate that the approach is viable. We show that the instance-based learner operating on structured, relational data outperforms a propositional k-NN algorithm. In qualitative terms, some of the piano performances produced by DISTALL after learning from the human artist are of substantial musical quality; one even won a prize in an international 'computer music performance' contest. The experiments thus provide evidence of the capabilities of ILP in a highly complex domain such as music. |
---|
C02 | 01 | X | | @0 001D02C02 |
---|
C02 | 02 | X | | @0 001D02B07D |
---|
C03 | 01 | X | FRE | @0 Algorithme apprentissage @5 01 |
---|
C03 | 01 | X | ENG | @0 Learning algorithm @5 01 |
---|
C03 | 01 | X | SPA | @0 Algoritmo aprendizaje @5 01 |
---|
C03 | 02 | X | FRE | @0 Musique @5 02 |
---|
C03 | 02 | X | ENG | @0 Music @5 02 |
---|
C03 | 02 | X | SPA | @0 Música @5 02 |
---|
C03 | 03 | X | FRE | @0 Structure donnée @5 03 |
---|
C03 | 03 | X | ENG | @0 Data structure @5 03 |
---|
C03 | 03 | X | SPA | @0 Estructura datos @5 03 |
---|
C03 | 04 | X | FRE | @0 Base donnée relationnelle @5 04 |
---|
C03 | 04 | X | ENG | @0 Relational database @5 04 |
---|
C03 | 04 | X | SPA | @0 Base relacional dato @5 04 |
---|
C03 | 05 | X | FRE | @0 Fouille donnée @5 05 |
---|
C03 | 05 | X | ENG | @0 Data mining @5 05 |
---|
C03 | 05 | X | SPA | @0 Busca dato @5 05 |
---|
C03 | 06 | X | FRE | @0 Extraction information @5 06 |
---|
C03 | 06 | X | ENG | @0 Information extraction @5 06 |
---|
C03 | 06 | X | SPA | @0 Extracción información @5 06 |
---|
C03 | 07 | X | FRE | @0 Analyse donnée @5 07 |
---|
C03 | 07 | X | ENG | @0 Data analysis @5 07 |
---|
C03 | 07 | X | SPA | @0 Análisis datos @5 07 |
---|
C03 | 08 | 3 | FRE | @0 Programmation logique inductive @5 08 |
---|
C03 | 08 | 3 | ENG | @0 Inductive logic programming @5 08 |
---|
C03 | 09 | X | FRE | @0 Apprentissage basé instance relationnelle @4 INC @5 82 |
---|
N21 | | | | @1 289 |
---|
N44 | 01 | | | @1 PSI |
---|
N82 | | | | @1 PSI |
---|
|
pR |
A30 | 01 | 1 | ENG | @1 International Conference on Inductive Logic Programming (IPL 2003) @2 13 @3 Szeged HUN @4 2003-09-29 |
---|
|
Format Inist (serveur)
NO : | PASCAL 06-0438222 INIST |
ET : | Relational IBL in classical music |
AU : | TOBUDIC (Asmir); WIDMER (Gerhard) |
AF : | Austrian Research Institute for Artificial Intelligence/Vienna/Autriche (1 aut.); Department of Computational Perception, Johannes Kepler University Linz, and Austrian Research Institute for Artificial Intelligence/Vienna/Autriche (2 aut.) |
DT : | Publication en série; Congrès; Niveau analytique |
SO : | Machine learning; ISSN 0885-6125; Pays-Bas; Da. 2006; Vol. 64; No. 1-3; Pp. 5-24; Bibl. 19 ref. |
LA : | Anglais |
EA : | It is well known that many hard tasks considered in machine learning and data mining can be solved in a rather simple and robust way with an instance- and distance-based approach. In this work we present another difficult task: learning, from large numbers of complex performances by concert pianists, to play music expressively. We model the problem as a multi-level decomposition and prediction task. We show that this is a fundamentally relational learning problem and propose a new similarity measure for structured objects, which is built into a relational instance-based learning algorithm named DISTALL. Experiments with data derived from a substantial number of Mozart piano sonata recordings by a skilled concert pianist demonstrate that the approach is viable. We show that the instance-based learner operating on structured, relational data outperforms a propositional k-NN algorithm. In qualitative terms, some of the piano performances produced by DISTALL after learning from the human artist are of substantial musical quality; one even won a prize in an international 'computer music performance' contest. The experiments thus provide evidence of the capabilities of ILP in a highly complex domain such as music. |
CC : | 001D02C02; 001D02B07D |
FD : | Algorithme apprentissage; Musique; Structure donnée; Base donnée relationnelle; Fouille donnée; Extraction information; Analyse donnée; Programmation logique inductive; Apprentissage basé instance relationnelle |
ED : | Learning algorithm; Music; Data structure; Relational database; Data mining; Information extraction; Data analysis; Inductive logic programming |
SD : | Algoritmo aprendizaje; Música; Estructura datos; Base relacional dato; Busca dato; Extracción información; Análisis datos |
LO : | INIST-21011.354000133342720010 |
ID : | 06-0438222 |
Links to Exploration step
Pascal:06-0438222
Le document en format XML
<record><TEI><teiHeader><fileDesc><titleStmt><title xml:lang="en" level="a">Relational IBL in classical music</title>
<author><name sortKey="Tobudic, Asmir" sort="Tobudic, Asmir" uniqKey="Tobudic A" first="Asmir" last="Tobudic">Asmir Tobudic</name>
<affiliation><inist:fA14 i1="01"><s1>Austrian Research Institute for Artificial Intelligence</s1>
<s2>Vienna</s2>
<s3>AUT</s3>
<sZ>1 aut.</sZ>
</inist:fA14>
</affiliation>
</author>
<author><name sortKey="Widmer, Gerhard" sort="Widmer, Gerhard" uniqKey="Widmer G" first="Gerhard" last="Widmer">Gerhard Widmer</name>
<affiliation><inist:fA14 i1="02"><s1>Department of Computational Perception, Johannes Kepler University Linz, and Austrian Research Institute for Artificial Intelligence</s1>
<s2>Vienna</s2>
<s3>AUT</s3>
<sZ>2 aut.</sZ>
</inist:fA14>
</affiliation>
</author>
</titleStmt>
<publicationStmt><idno type="wicri:source">INIST</idno>
<idno type="inist">06-0438222</idno>
<date when="2006">2006</date>
<idno type="stanalyst">PASCAL 06-0438222 INIST</idno>
<idno type="RBID">Pascal:06-0438222</idno>
<idno type="wicri:Area/PascalFrancis/Corpus">000151</idno>
</publicationStmt>
<sourceDesc><biblStruct><analytic><title xml:lang="en" level="a">Relational IBL in classical music</title>
<author><name sortKey="Tobudic, Asmir" sort="Tobudic, Asmir" uniqKey="Tobudic A" first="Asmir" last="Tobudic">Asmir Tobudic</name>
<affiliation><inist:fA14 i1="01"><s1>Austrian Research Institute for Artificial Intelligence</s1>
<s2>Vienna</s2>
<s3>AUT</s3>
<sZ>1 aut.</sZ>
</inist:fA14>
</affiliation>
</author>
<author><name sortKey="Widmer, Gerhard" sort="Widmer, Gerhard" uniqKey="Widmer G" first="Gerhard" last="Widmer">Gerhard Widmer</name>
<affiliation><inist:fA14 i1="02"><s1>Department of Computational Perception, Johannes Kepler University Linz, and Austrian Research Institute for Artificial Intelligence</s1>
<s2>Vienna</s2>
<s3>AUT</s3>
<sZ>2 aut.</sZ>
</inist:fA14>
</affiliation>
</author>
</analytic>
<series><title level="j" type="main">Machine learning</title>
<title level="j" type="abbreviated">Mach. learn.</title>
<idno type="ISSN">0885-6125</idno>
<imprint><date when="2006">2006</date>
</imprint>
</series>
</biblStruct>
</sourceDesc>
<seriesStmt><title level="j" type="main">Machine learning</title>
<title level="j" type="abbreviated">Mach. learn.</title>
<idno type="ISSN">0885-6125</idno>
</seriesStmt>
</fileDesc>
<profileDesc><textClass><keywords scheme="KwdEn" xml:lang="en"><term>Data analysis</term>
<term>Data mining</term>
<term>Data structure</term>
<term>Inductive logic programming</term>
<term>Information extraction</term>
<term>Learning algorithm</term>
<term>Music</term>
<term>Relational database</term>
</keywords>
<keywords scheme="Pascal" xml:lang="fr"><term>Algorithme apprentissage</term>
<term>Musique</term>
<term>Structure donnée</term>
<term>Base donnée relationnelle</term>
<term>Fouille donnée</term>
<term>Extraction information</term>
<term>Analyse donnée</term>
<term>Programmation logique inductive</term>
<term>Apprentissage basé instance relationnelle</term>
</keywords>
</textClass>
</profileDesc>
</teiHeader>
<front><div type="abstract" xml:lang="en">It is well known that many hard tasks considered in machine learning and data mining can be solved in a rather simple and robust way with an instance- and distance-based approach. In this work we present another difficult task: learning, from large numbers of complex performances by concert pianists, to play music expressively. We model the problem as a multi-level decomposition and prediction task. We show that this is a fundamentally relational learning problem and propose a new similarity measure for structured objects, which is built into a relational instance-based learning algorithm named DISTALL. Experiments with data derived from a substantial number of Mozart piano sonata recordings by a skilled concert pianist demonstrate that the approach is viable. We show that the instance-based learner operating on structured, relational data outperforms a propositional k-NN algorithm. In qualitative terms, some of the piano performances produced by DISTALL after learning from the human artist are of substantial musical quality; one even won a prize in an international 'computer music performance' contest. The experiments thus provide evidence of the capabilities of ILP in a highly complex domain such as music.</div>
</front>
</TEI>
<inist><standard h6="B"><pA><fA01 i1="01" i2="1"><s0>0885-6125</s0>
</fA01>
<fA03 i2="1"><s0>Mach. learn.</s0>
</fA03>
<fA06><s2>1-3</s2>
</fA06>
<fA08 i1="01" i2="1" l="ENG"><s1>Relational IBL in classical music</s1>
</fA08>
<fA11 i1="01" i2="1"><s1>TOBUDIC (Asmir)</s1>
</fA11>
<fA11 i1="02" i2="1"><s1>WIDMER (Gerhard)</s1>
</fA11>
<fA14 i1="01"><s1>Austrian Research Institute for Artificial Intelligence</s1>
<s2>Vienna</s2>
<s3>AUT</s3>
<sZ>1 aut.</sZ>
</fA14>
<fA14 i1="02"><s1>Department of Computational Perception, Johannes Kepler University Linz, and Austrian Research Institute for Artificial Intelligence</s1>
<s2>Vienna</s2>
<s3>AUT</s3>
<sZ>2 aut.</sZ>
</fA14>
<fA20><s1>5-24</s1>
</fA20>
<fA21><s1>2006</s1>
</fA21>
<fA23 i1="01"><s0>ENG</s0>
</fA23>
<fA43 i1="01"><s1>INIST</s1>
<s2>21011</s2>
<s5>354000133342720010</s5>
</fA43>
<fA44><s0>0000</s0>
<s1>© 2006 INIST-CNRS. All rights reserved.</s1>
</fA44>
<fA45><s0>19 ref.</s0>
</fA45>
<fA47 i1="01" i2="1"><s0>06-0438222</s0>
</fA47>
<fA60><s1>P</s1>
<s2>C</s2>
</fA60>
<fA64 i1="01" i2="1"><s0>Machine learning</s0>
</fA64>
<fA66 i1="01"><s0>NLD</s0>
</fA66>
<fC01 i1="01" l="ENG"><s0>It is well known that many hard tasks considered in machine learning and data mining can be solved in a rather simple and robust way with an instance- and distance-based approach. In this work we present another difficult task: learning, from large numbers of complex performances by concert pianists, to play music expressively. We model the problem as a multi-level decomposition and prediction task. We show that this is a fundamentally relational learning problem and propose a new similarity measure for structured objects, which is built into a relational instance-based learning algorithm named DISTALL. Experiments with data derived from a substantial number of Mozart piano sonata recordings by a skilled concert pianist demonstrate that the approach is viable. We show that the instance-based learner operating on structured, relational data outperforms a propositional k-NN algorithm. In qualitative terms, some of the piano performances produced by DISTALL after learning from the human artist are of substantial musical quality; one even won a prize in an international 'computer music performance' contest. The experiments thus provide evidence of the capabilities of ILP in a highly complex domain such as music.</s0>
</fC01>
<fC02 i1="01" i2="X"><s0>001D02C02</s0>
</fC02>
<fC02 i1="02" i2="X"><s0>001D02B07D</s0>
</fC02>
<fC03 i1="01" i2="X" l="FRE"><s0>Algorithme apprentissage</s0>
<s5>01</s5>
</fC03>
<fC03 i1="01" i2="X" l="ENG"><s0>Learning algorithm</s0>
<s5>01</s5>
</fC03>
<fC03 i1="01" i2="X" l="SPA"><s0>Algoritmo aprendizaje</s0>
<s5>01</s5>
</fC03>
<fC03 i1="02" i2="X" l="FRE"><s0>Musique</s0>
<s5>02</s5>
</fC03>
<fC03 i1="02" i2="X" l="ENG"><s0>Music</s0>
<s5>02</s5>
</fC03>
<fC03 i1="02" i2="X" l="SPA"><s0>Música</s0>
<s5>02</s5>
</fC03>
<fC03 i1="03" i2="X" l="FRE"><s0>Structure donnée</s0>
<s5>03</s5>
</fC03>
<fC03 i1="03" i2="X" l="ENG"><s0>Data structure</s0>
<s5>03</s5>
</fC03>
<fC03 i1="03" i2="X" l="SPA"><s0>Estructura datos</s0>
<s5>03</s5>
</fC03>
<fC03 i1="04" i2="X" l="FRE"><s0>Base donnée relationnelle</s0>
<s5>04</s5>
</fC03>
<fC03 i1="04" i2="X" l="ENG"><s0>Relational database</s0>
<s5>04</s5>
</fC03>
<fC03 i1="04" i2="X" l="SPA"><s0>Base relacional dato</s0>
<s5>04</s5>
</fC03>
<fC03 i1="05" i2="X" l="FRE"><s0>Fouille donnée</s0>
<s5>05</s5>
</fC03>
<fC03 i1="05" i2="X" l="ENG"><s0>Data mining</s0>
<s5>05</s5>
</fC03>
<fC03 i1="05" i2="X" l="SPA"><s0>Busca dato</s0>
<s5>05</s5>
</fC03>
<fC03 i1="06" i2="X" l="FRE"><s0>Extraction information</s0>
<s5>06</s5>
</fC03>
<fC03 i1="06" i2="X" l="ENG"><s0>Information extraction</s0>
<s5>06</s5>
</fC03>
<fC03 i1="06" i2="X" l="SPA"><s0>Extracción información</s0>
<s5>06</s5>
</fC03>
<fC03 i1="07" i2="X" l="FRE"><s0>Analyse donnée</s0>
<s5>07</s5>
</fC03>
<fC03 i1="07" i2="X" l="ENG"><s0>Data analysis</s0>
<s5>07</s5>
</fC03>
<fC03 i1="07" i2="X" l="SPA"><s0>Análisis datos</s0>
<s5>07</s5>
</fC03>
<fC03 i1="08" i2="3" l="FRE"><s0>Programmation logique inductive</s0>
<s5>08</s5>
</fC03>
<fC03 i1="08" i2="3" l="ENG"><s0>Inductive logic programming</s0>
<s5>08</s5>
</fC03>
<fC03 i1="09" i2="X" l="FRE"><s0>Apprentissage basé instance relationnelle</s0>
<s4>INC</s4>
<s5>82</s5>
</fC03>
<fN21><s1>289</s1>
</fN21>
<fN44 i1="01"><s1>PSI</s1>
</fN44>
<fN82><s1>PSI</s1>
</fN82>
</pA>
<pR><fA30 i1="01" i2="1" l="ENG"><s1>International Conference on Inductive Logic Programming (IPL 2003)</s1>
<s2>13</s2>
<s3>Szeged HUN</s3>
<s4>2003-09-29</s4>
</fA30>
</pR>
</standard>
<server><NO>PASCAL 06-0438222 INIST</NO>
<ET>Relational IBL in classical music</ET>
<AU>TOBUDIC (Asmir); WIDMER (Gerhard)</AU>
<AF>Austrian Research Institute for Artificial Intelligence/Vienna/Autriche (1 aut.); Department of Computational Perception, Johannes Kepler University Linz, and Austrian Research Institute for Artificial Intelligence/Vienna/Autriche (2 aut.)</AF>
<DT>Publication en série; Congrès; Niveau analytique</DT>
<SO>Machine learning; ISSN 0885-6125; Pays-Bas; Da. 2006; Vol. 64; No. 1-3; Pp. 5-24; Bibl. 19 ref.</SO>
<LA>Anglais</LA>
<EA>It is well known that many hard tasks considered in machine learning and data mining can be solved in a rather simple and robust way with an instance- and distance-based approach. In this work we present another difficult task: learning, from large numbers of complex performances by concert pianists, to play music expressively. We model the problem as a multi-level decomposition and prediction task. We show that this is a fundamentally relational learning problem and propose a new similarity measure for structured objects, which is built into a relational instance-based learning algorithm named DISTALL. Experiments with data derived from a substantial number of Mozart piano sonata recordings by a skilled concert pianist demonstrate that the approach is viable. We show that the instance-based learner operating on structured, relational data outperforms a propositional k-NN algorithm. In qualitative terms, some of the piano performances produced by DISTALL after learning from the human artist are of substantial musical quality; one even won a prize in an international 'computer music performance' contest. The experiments thus provide evidence of the capabilities of ILP in a highly complex domain such as music.</EA>
<CC>001D02C02; 001D02B07D</CC>
<FD>Algorithme apprentissage; Musique; Structure donnée; Base donnée relationnelle; Fouille donnée; Extraction information; Analyse donnée; Programmation logique inductive; Apprentissage basé instance relationnelle</FD>
<ED>Learning algorithm; Music; Data structure; Relational database; Data mining; Information extraction; Data analysis; Inductive logic programming</ED>
<SD>Algoritmo aprendizaje; Música; Estructura datos; Base relacional dato; Busca dato; Extracción información; Análisis datos</SD>
<LO>INIST-21011.354000133342720010</LO>
<ID>06-0438222</ID>
</server>
</inist>
</record>
Pour manipuler ce document sous Unix (Dilib)
EXPLOR_STEP=$WICRI_ROOT/Wicri/Musique/explor/MozartV1/Data/PascalFrancis/Corpus
HfdSelect -h $EXPLOR_STEP/biblio.hfd -nk 000151 | SxmlIndent | more
Ou
HfdSelect -h $EXPLOR_AREA/Data/PascalFrancis/Corpus/biblio.hfd -nk 000151 | SxmlIndent | more
Pour mettre un lien sur cette page dans le réseau Wicri
{{Explor lien
|wiki= Wicri/Musique
|area= MozartV1
|flux= PascalFrancis
|étape= Corpus
|type= RBID
|clé= Pascal:06-0438222
|texte= Relational IBL in classical music
}}
| This area was generated with Dilib version V0.6.20. Data generation: Sun Apr 10 15:06:14 2016. Site generation: Tue Feb 7 15:40:35 2023 | 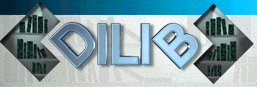 |